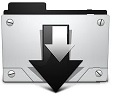

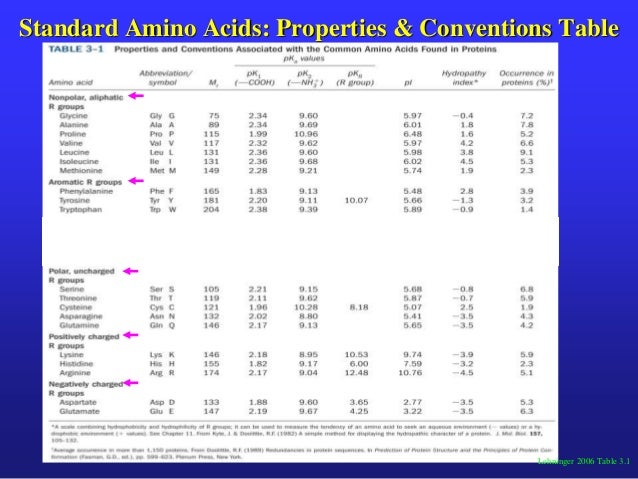
These results suggest that factor mixture modeling is sufficiently robust for detecting heterogeneous populations even when group mean differences are modest.įertility and testicular function were studied in eight men with severe homozygous (Pi ZZ variant genotype) alpha-1 antitrypsin (AAT) deficiency. 49, which is significantly greater than heritability for the rest of the population in early childhood. Model-fitting analyses estimated group heritability as. Simulation results based on variance components derived from Turkheimer and colleagues (2003), illustrate the impact of factors such as the difference in group means and variance components on the feasibility of correctly estimating the parameters of the mixture model. Various models for the class membership of twin pairs may be employed, including additive genetic, common environment, specific environment or major locus (QTL) factors. This model may be considered as a special case of a factor mixture model, which combines the features of a latent class model with those of a latent trait model. We therefore present a finite mixture model which specifies that the population consists of latent groups which may differ in (i) their means, and (ii) the relative impact of genetic and environmental factors on within-group variation and covariation. While useful for detecting such effects, formal modeling of differences in variance components as a function of pairs' trait scores is rarely attempted. An intriguing finding was the presence of significant differences in E1 variance for different PI types, suggesting that different phenotypes have differing capacities to react to environmental challenges.Īpproaches such as DeFries-Fulker extremes regression (LaBuda et al., 1986) are commonly used in genetically informative studies to assess whether familial resemblance varies as a function of the scores of pairs of twins. 63, which may reflect, in part, the rather low reliability of the assays and account for the modest heritabilities (less than.
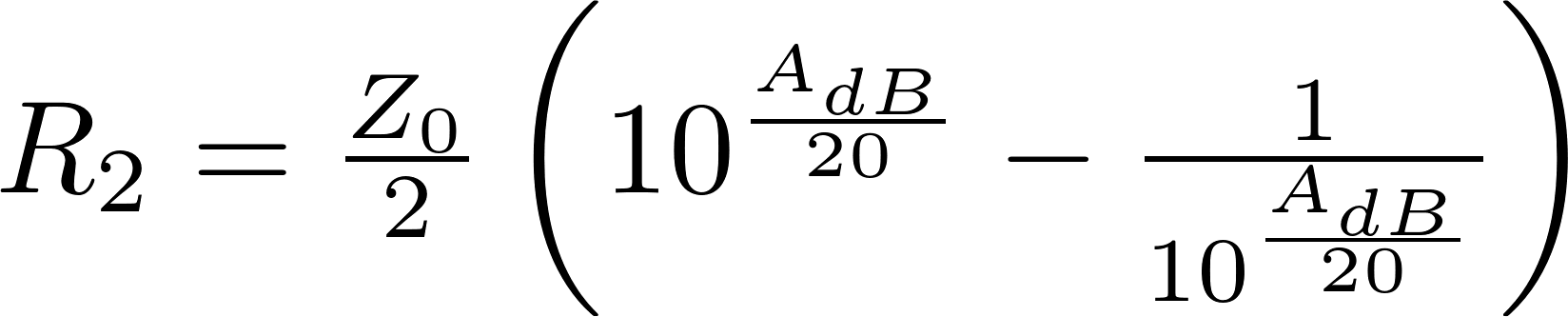
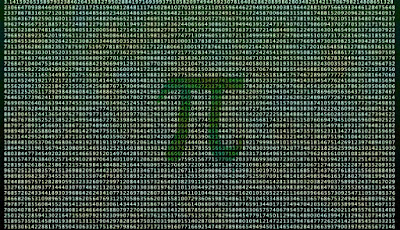
The correlation between environmental deviations for the two measures was only. Bivariate analysis of alpha-1-antitrypsin and EIC revealed marginal evidence for differences in specific activities of molecules coded by different PI alleles. We conclude that nearly all genetic variation in alpha-1-antitrypsin quantity and activity can be explained by detectable variation at the PI locus and that this variance is largely additive. Only small improvements in fit could then be obtained by addition of polygenic components of variance. The 29 phenotype means could also be described as the appropriate additive combinations of the 12 allelic effects. Specification of phenotypic means led to highly significant improvements in fit over models including only individual environment variance and additive genetic variance. Models of variation were fitted directly to the raw observations by a maximum-likelihood method. We have measured the quantity of alpha-1-antitrypsin (by an immunoturbidometric assay) and its activity (by assaying elastase inhibitory capacity ) in 583 individuals from 114 twin kinships who were also typed for PI by isoelectric focusing. Whether genetic factors other than the protease-inhibitor (PI) polymorphism itself contribute to variation in alpha-1-antitrypsin is of both theoretical and practical interest.
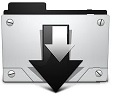